Ratiometric SERS imaging for indication of peroxynitrite fluctuations in diabetic wound healing process
Author links open overlay panel,
,
,
,
,
- a
- Key Laboratory of Hainan Trauma and Disaster Rescue, The First Affiliated Hospital of Hainan Medical University, Hainan Medical University, Haikou 571199, China
- b
- Engineering Research Center for Hainan Bio-Smart Materials and Bio-Medical Devices, Key Laboratory of Emergency and Trauma, Ministry of Education, Key Laboratory of Hainan Functional Materials and Molecular Imaging, College of Emergency and Trauma, Hainan Medical University, Haikou 571199, China
Received 27 March 2023, Revised 13 May 2023, Accepted 7 June 2023, Available online 8 June 2023, Version of Record 19 June 2023.
https://doi.org/10.1016/j.cej.2023.144024
Highlights
•
SERS nanoprobes were prepared through the Au@Ag core-ultrathin shell nanoparticles.
•
SERS nanoprobes could sensitively detect ONOO− in solution and living cells.
•
Dynamic fluctuations of ONOO− could be detected during the wound healing process
Abstract
Peroxynitrite (ONOO−) involves in diabetic wounds and its concentration is fluctuated in different pathological stages during the wound healing process. Thus, ONOO− may be utilized as a powerful biomarker to indicate the healing process of diabetic wound. However, the pathological roles of ONOO− can’t be fully understood yet. Herein, inspired by the mechanism that phenylboronic pinacol ester can react with ONOO− specifically, we report a new kind of SERS nanoprobes to monitor ONOO− fluctuation during diabetic wound healing process. The gold-silver (Au@Ag) core-ultrathin shell nanoparticles were utilized as active substrate and conjugated with phenylboronic pinacol ester molecules on the surface of Ag shell to simultaneously act as Raman reporter molecules and recognition moiety of ONOO−. In addition, functional polyethylene glycol (HS-PEG) and bovine serum albumin layers were sequentially immobilized on the surface of Ag shell to improve the stability of the nanoprobes. In the presence of ONOO−, phenylboronic pinacol ester could be converted into the corresponding phenol, inducing the SERS signal changes. The concentration fluctuation of ONOO− could be quantitatively detected through the SERS signal intensity ratio (1076 cm−1/998 cm−1) changes of typical peaks. The nanoprobes exhibited a wide response range from 0 to 75 μM with 0.12 μM as the detection limit. In the diabetic wound skin models, ONOO− concentration fluctuations during the wound healing process before and after drug treatment could be obtained by SERS imaging. We expect the proposed SERS imaging assay could be a noninvasive and powerful tool to monitor the diabetic wound healing.
Keywords
Surface-enhanced Raman scattering (SERS) imaging
Peroxynitrite fluctuation
Diabetes mellitus
Wound healing
Living cell and in vivo analysis
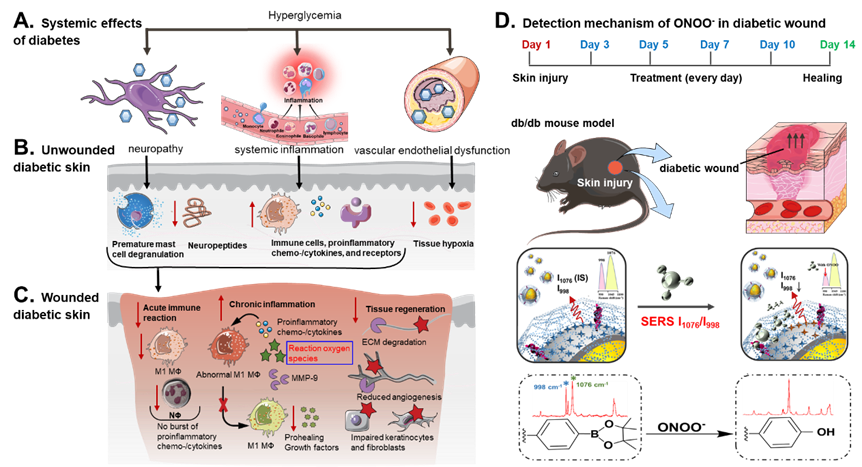
Scheme 1. Pathophysiology of diabetic wound healing and SERS detection of ONOO− for diabetic wound. (A) Systemic and (B) local diabetes-related physiological alternations in immune/ inflammatory response, cell/tissue function. (C) The reduced inflammatory response upon injury induces the chronic inflammation and lower tissue regeneration, especially the reaction oxygen species burst. (D) SERS detection of ONOO− in diabetic wound for healing monitoring. MФ, macrophage. The illustrations were created with the help of BioRender.com.
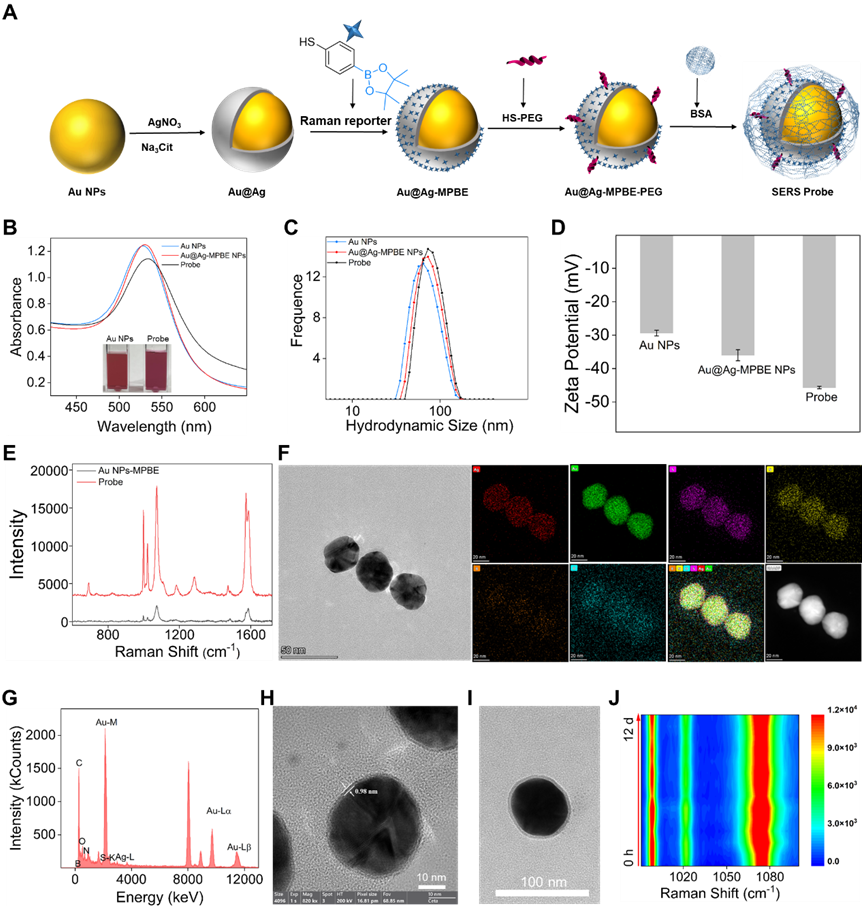
Fig. 1. Preparation and characterization of SERS probes. (A) The preparation protocol of SERS probes. (B) UV–vis spectra of SERS probe and precursor nanoparticles, insert: photographs of Au NPs and probes. (C) DLS distributions. (D) Zeta potential. (E) SERS spectra of Au NPs and probes. (F-G) HAADF-STEM and EDS elemental maps of SERS probes. (H) TEM image of Au@Ag NPs displays the ultrathin silver shell. (J) TEM image of probe indicates the BSA shell. (J) Stability test of SERS signal intensity of SERS probes within 12 days.
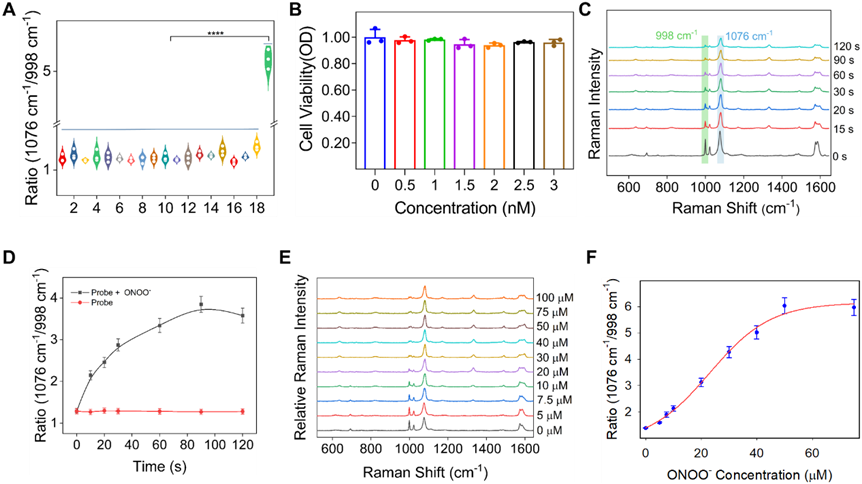
Fig. 2. (A) Raman intensity ratio (I1076/I998) of SERS probe (1 nM) in the presence of various ROS, RNS and other analytes within 3 min. 1. PBS control; 2. MgSO4; 3. CuSO4; 4. KCl; 5. Na2CO3; 6. Na2SO4; 7. Na2S; 8. CaCl2; 9. ZnSO4; 10. NaHSO3; 11. GSH; 12·H2O2; 13. ClO−; 14. AchE; 15. O2
−; 16. NO; 17. OH− (2–17 100 μM); 18. L-Cys (300 μM); 19. ONOO− (50 μM). (B) Cell viability of the SERS probe against RAW 264.7 cells. (C) Time-dependent SERS spectra and corresponding Raman intensity distribution at I1076/I998 of the probe toward ONOO− (50 μM) acquired at 2 min. (D) Raman intensity ratio of I1076/I998 corresponding to (C). (E) Changes of the Raman intensity of probe (1 nM) incubated with an increasing concentration of ONOO− (0–100 μM). (F) Corresponding calibration line of Raman intensity ratio of I1076/I998 as a function of the sigmoidal of the concentration of ONOO−. All spectra were measured in PBS containing 20% FBS (20 mM, pH 7.4, v/v, 633 nm-laser power, 1 s exposure time). Statistical analysis was performed using one-way analysis of variance followed by post hoc Tukey multiple comparisons (n = 3, mean ± S. E. M., ***P < 0.001).
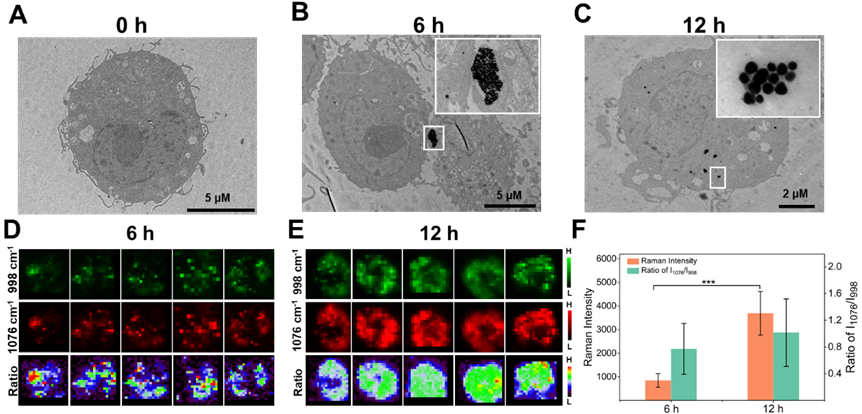
Fig. 3. (A–C) TEM images of RAW 264.7 cells incubated with probe (1 nM) for 0 h, 6 h and 12 h, respectively. (D) and (E) Intracellular SERS mapping images of five random RAW 264.7 cells incubated with probe (1 nM) for 6 h and 12 h, respectively. (F) Mean Raman signal intensity and intensity ratio (I1076/I998) of five random cells in (D) and (E). Single-cell SERS mapping was achieved using exposure time of 0.5 s (633 nm-laser power, 50 × objective). Statistical analysis was performed using one-way analysis of variance followed by post hoc Tukey multiple comparisons (n = 5, mean ± S. E. M., ***P < 0.001).
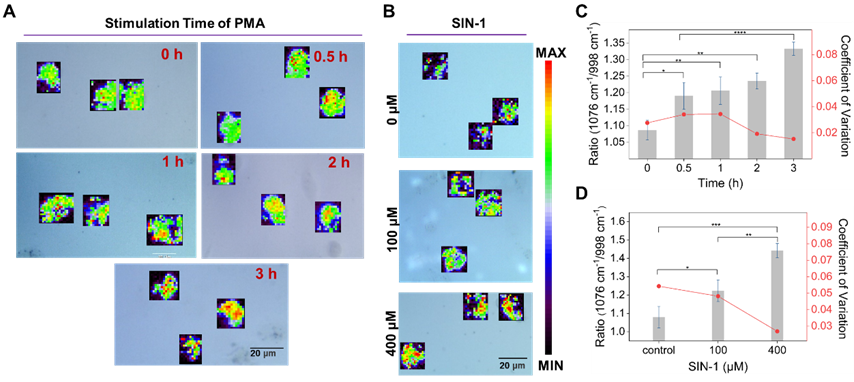
Fig. 4. (A) SERS mapping images after stimulation with PMA (10 nM) for 0 h, 0.5 h, 1 h, 2 h and 3 h, respectively. (B) SERS mapping images after pretreatment with different concentrations of ONOO− donor SIN-1 (0, 100 and 400 μM). (C) and (D) Statistical analysis of Raman intensity ratio of I1076/I998 in (A) and (B), respectively. Single-cell SERS mapping was achieved using exposure time of 0.5 s (633 nm-laser power, 50 × objective). Statistical analysis was performed using one-way analysis of variance followed by post hoc Tukey multiple comparisons (n = 3, mean ± S. E. M., *P < 0.05, **P < 0.01, ***P < 0.001, ****P < 0.0001).
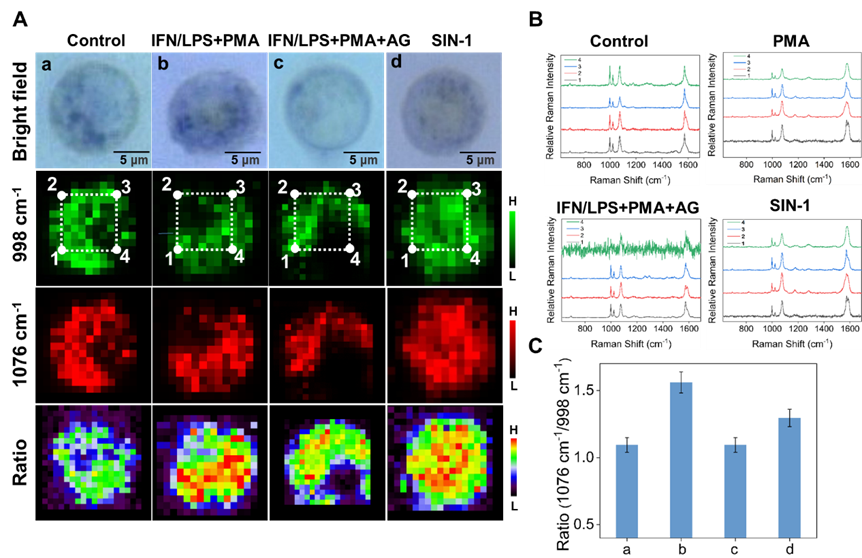
Fig. 5. (A) Single-cell SERS mapping images of RAW 264.7 cells. These cells were pretreated with the probe (1 nM) for 12 h. (a) PBS control group; (b) cells were incubated with LPS (1 μg/mL) and IFN-γ (50 ng/mL) for 4 h and then PMA (10 nM) for 3 h in the culture medium; (c) cells were pretreated with LPS (1 μg/mL) and IFN-γ (50 ng/mL) for 4 h, then PMA (10 nM) for 3 h and AG (1 mM) for 30 min in the culture medium; (d) cells were incubated with ONOO– donor SIN-1 (400 μM) for 2 h. (B) SERS spectra of the different positions in (A). (C) Quantification of Raman signal intensity ratio of I1076/I998 in (A). Single-cell SERS mapping was achieved using exposure time of 0.5 s (633 nm-laser power, 50 × objective).
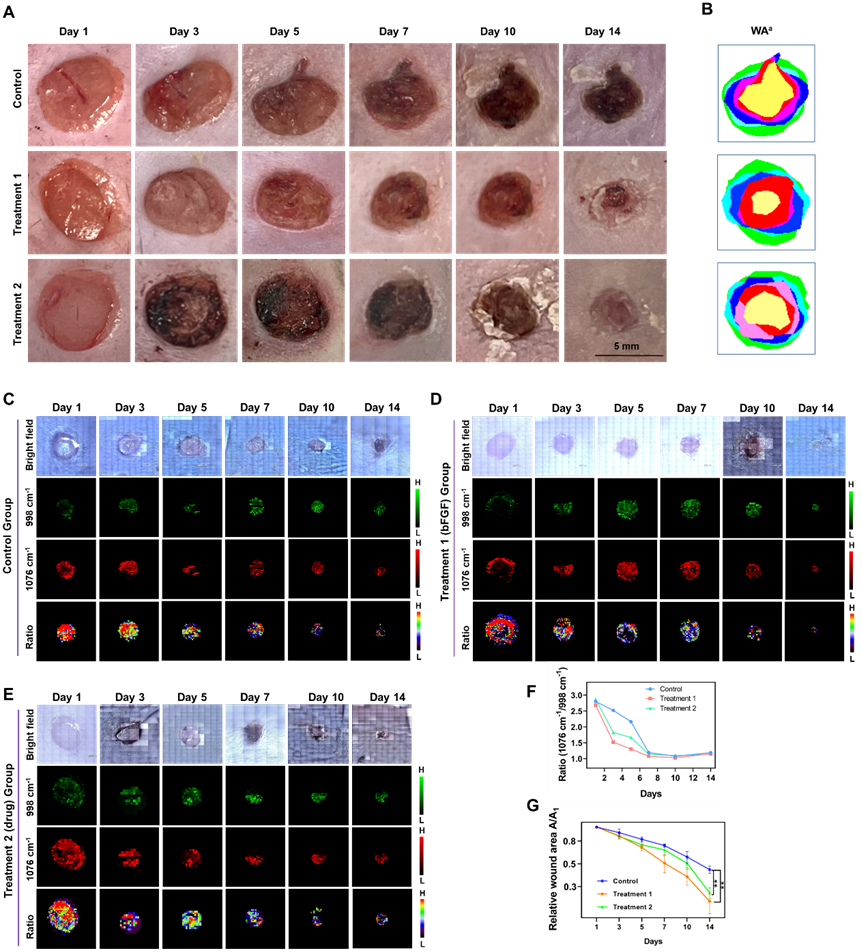
Fig. 6. (A) Representative photographic images of db/db mice with wounds in the groups of the untreated control, or treated with bFGF (treatment 1 group) and metformin + empagliflozin (treatment 2 group). (B) Simulation images of wound area in different groups. The green area meant the initial area and yellow area represented the wound area at 14 day. (C) - (E) Corresponding pseudo-color-coded in vivo SERS mapping images of the probe-applied wounds at up to 14 days without and with treatment. (F) and (G) Quantification of SERS intensity ratio and wound area of diabetic wounds after applying the probe in the control group, treatment 1 group and treatment 2 group, respectively. Wound SERS mapping was achieved using exposure time of 0.5 s (633 nm-laser power, 50 × objective). Statistical analysis was performed using one-way analysis of variance followed by post hoc Tukey multiple comparisons (n = 3, mean ± S. E. M., **P < 0.01).
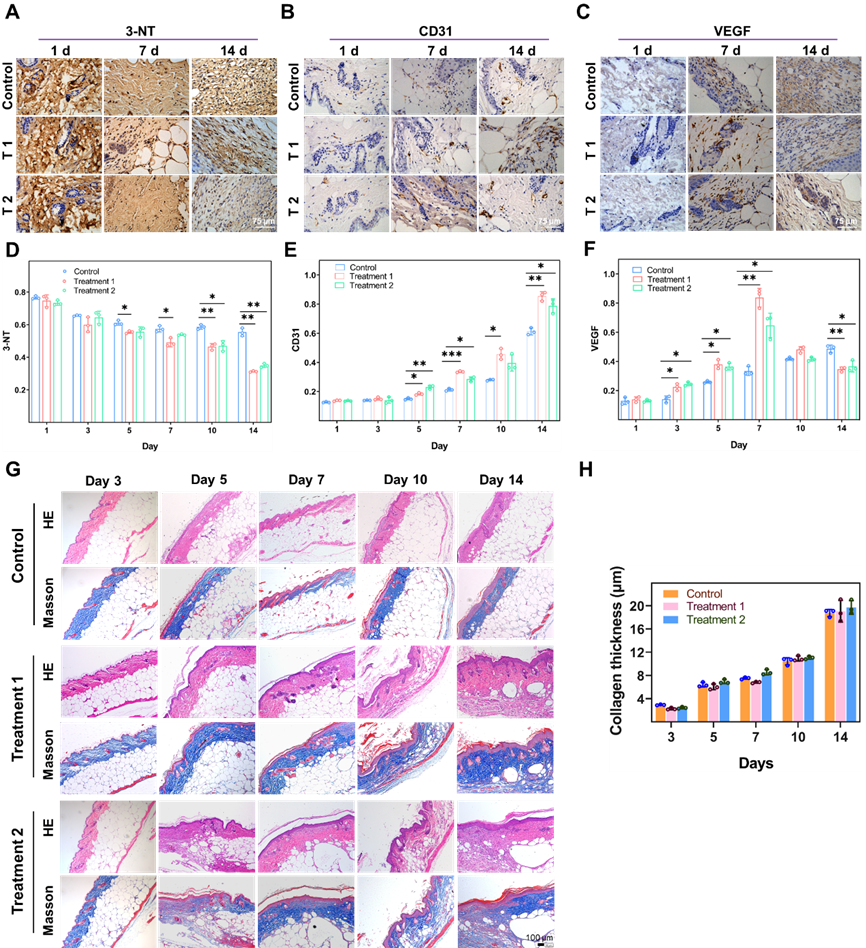
Fig. 7. Immunohistochemistry images of 3-NT (A), CD31 (B) and VEGF (C) of wounded skin tissues with and without treatment at different time points. Scale bar is 75 μm. (D) – (F) Quantification of immunohistochemistry images corresponding to (A) – (C). Three random view fields were selected under 40 × microscope and Image J software was used to calculate and count the mean OD values as the number of positive cells. (G) H&E and Masson staining of wounded skin tissues with and without treatment at different time points. (H) Quantification of Masson staining images of (G). Statistical analysis was performed using one-way analysis of variance followed by post hoc Tukey multiple comparisons (n = 3, mean ± S. E. M., *P < 0.05, **P < 0.01, ***P < 0.001).
4. Conclusion
In summary, we explored a SERS imaging method using sensitive SERS nanoprobes for indication of ONOO− fluctuations at the single cell level and during the healing process of diabetic wound. Au@Ag core-ultrathin shell nanoparticles immobilizing phenylboronic pinacol ester were embedded with HS-PEG and BSA on the surface of Ag shell to achieve sensitive detection of ONOO− at single cell level and diabetic wound skin models. These results demonstrated that the proposed SERS nanoprobes exhibited high sensitivity, specificity and stability in the biological milieu. We believe that the detection of ONOO− fluctuations could broaden the applications of SERS imaging in biomedical research.